What is NMT
With the rapid development of deep learning technology, Neural Machine Translation (NMT) has made significant progress in language pairs with large parallel corpora. However, for low-resource languages that lack data, the performance of NMT models tends to drop significantly.
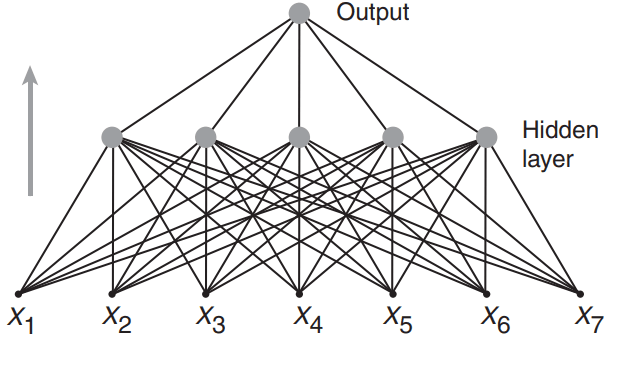
Challenges of Low-Resource NMT
The main challenges faced by low-resource NMT are:
- Data scarcity: Low-resource languages suffer from a lack of high-quality parallel corpora, which limits the training scale and performance of NMT models.
- Language diversity: There are differences in grammar, vocabulary, and semantics between different languages, which makes it difficult for NMT models to learn.
- Resource scarcity: Low-resource languages often lack researchers and funding, which hinders the development of related technologies.
- Data Quality and Quantity: NMT models require large amounts of high-quality parallel data (pairs of sentences in both source and target languages) to learn effectively. Obtaining such data can be challenging for languages with fewer available resources.
- Complexity of Language: Some languages have complex grammatical structures, idiomatic expressions, or ambiguous meanings, which can pose challenges for NMT models to accurately translate.
- Rare and Low-Resource Languages: For languages with limited digital resources or few speakers, building accurate NMT models is particularly difficult due to the lack of training data.
- Domain Adaptation: NMT models trained on general text may struggle to accurately translate specialized or domain-specific content, requiring additional adaptation.
Opportunities for Low-Resource NMT
Despite many challenges, low-resource NMT holds great promise for the future. The main reasons are as follows:
- Data growth: The普及of the internet and the development of information technology have made more and more data available, which provides new opportunities for low-resource NMT research.
- Algorithm improvement: NMT algorithms are constantly being improved, and models that can perform better with small amounts of data are being developed.
- Rise of multilingual learning: The rise of multilingual learning provides new思路for low-resource NMT research.
- Improved Translation Quality: NMT has shown significant improvements in translation quality compared to previous approaches, thanks to its ability to capture complex linguistic patterns.
- End-to-End Learning: NMT models learn to translate directly from input to output language, enabling smoother integration and potentially reducing errors introduced by intermediate steps.
- Continuous Improvement: NMT models can be continually refined and improved as more data becomes available or as the underlying algorithms evolve.
- Customization and Adaptation: NMT models can be fine-tuned or adapted to specific domains or styles, allowing for more accurate translations in specialized contexts.
Research Directions for Low-Resource NMT
Future research on low-resource NMT can focus on the following:
- Data augmentation: Explore new data augmentation methods to further improve the quality and diversity of training data.
- Model fusion: Research more effective model fusion methods to make full use of the advantages of each model.
- Multilingual learning: Utilize multilingual learning techniques to improve the generalization ability of low-resource NMT models.
- Research in specific scenarios: Develop low-resource NMT methods suitable for specific situations, such as multiple domains, morphologically rich languages, and distant language pairs.
Conclusion
Low-resource NMT is a challenging research task, but it also holds great potential. With future research, low-resource NMT technology is expected to develop further and contribute to improving the translation quality of low-resource languages.
Efficient and accurate real-time translation tool—Felo Translator

What is Felo Translator?
Felo Translator is an AI simultaneous interpretation app equipped with GPT-4 engine and RRT technology. It can quickly and accurately translate voice in more than 15 foreign languages (including English, Spanish, French, German, Russian, Chinese, Arabic, Japanese, etc.). It supports downloading original and translated text, helping you learn authentic expressions and pronunciation. ChatGPT, the large language model, accurately conveys the emotions, expressions, and dramatic effects of the play, enabling the audience to fully understand and enjoy the excitement brought by different language cultures.
How can Felo Translator assist simultaneous interpreters?
Felo Translator can assist beginners in simultaneous interpretation by solving the problem of falling behind in note-taking and ensuring more accurate translation of professional vocabulary.
Simultaneous interpretation is a complex and highly technical job that requires interpreters to have solid language skills, rich professional knowledge, and a good spirit of teamwork. Only by continuously learning and improving their translation abilities can they be competent in this important translation task and contribute to the smooth progress of international communication.
iOS Download | Android Download