Machine Translation Is More ‘Conservative’ Than Human Translation, Google Says
Googleが言うには、機械翻訳は人間の翻訳よりも「保守的」である
01
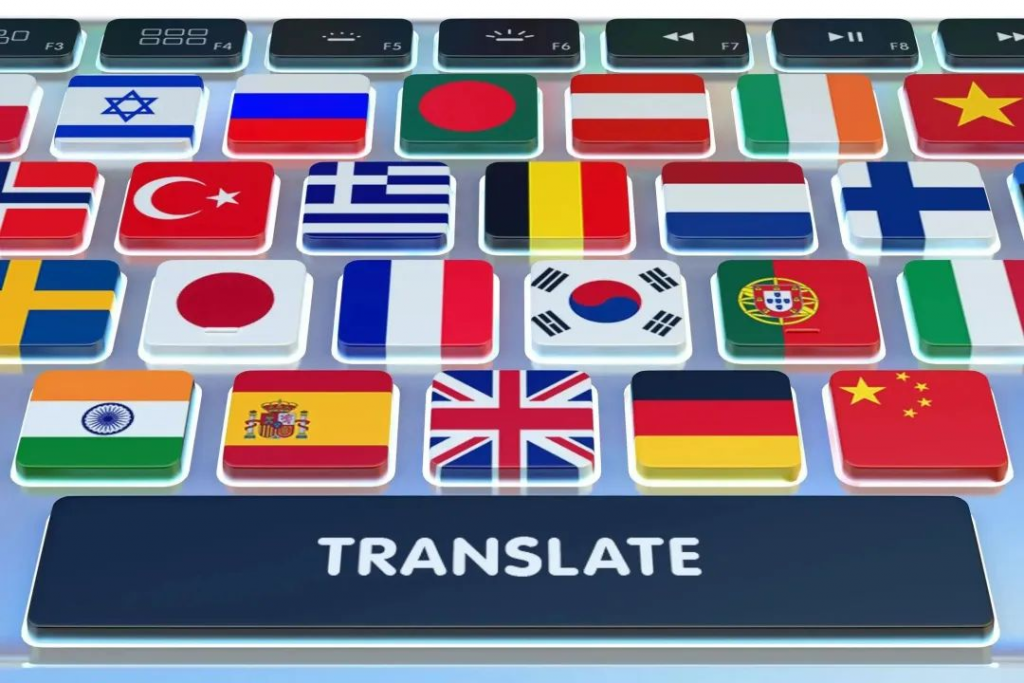
In a [paper] published on January 2, 2024, Google researchers Jiaming Luo, Colin Cherry, and George Foster compared morphosyntactic divergence in [machine translation] (MT) against human [translation] (HT) and found that MT tends to be more “conservative” than HT.
谷歌研究人员在2024年1月2日发表的論文中指出,与人工翻译相比,机器翻译更倾向于“保守”。研究人员Jiaming Luo、Colin Cherry和George Foster比较了机器翻译和人工翻译在形态句法上的差异,并发现机器翻译展现出较少的形态句法多样性,更多趋同模式,以及更多一对一的对齐。
The authors explained that translation divergences occur when translations differ structurally from source sentences. This can be due to inherent cross-lingual differences or idiosyncratic preferences of translators. These divergences naturally occur in the [translation] process and can be readily found in human translations — including those used for training MT systems — they said.
著者たちは、翻訳の相違は、翻訳が原文と構造的に異なる場合に生じると説明した。これは、固有の言語間の違いや翻訳者の特異な好みに起因する可能性がある。これらの相違は、翻訳プロセスで自然に発生し、人間の翻訳で容易に見つけることができると述べた。
They also highlighted that the existence of these divergences in HT has long been regarded as a key challenge for [MT], and recent studies have demonstrated the abundance of translation divergences in HT.
彼らはまた、HTでのこれらの相違の存在が長い間、[MT]の重要な課題と見なされてきたことを強調し、最近の研究で、HTでの翻訳の相違の豊富さが示されていると述べた。
To that end, they conducted experiments to assess how MT and HT differ in terms of morphosyntactic divergence, understand the source of this difference, and explore how translation divergences in HT affect MT quality. The experiments were conducted in three language pairs: English-French, English-German, and English-Chinese using WMT datasets.
このため、彼らは、形態句法の相違においてMTとHTがどのように異なるかを評価し、この違いの原因を理解し、HTの翻訳の相違がMTの品質にどのように影響するかを探るための実験を行った。実験は、WMTデータセットを使用して、英仏、英独、英中の3言語ペアで行われた。
02
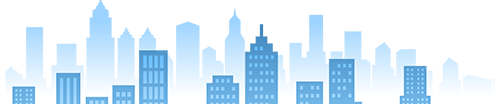
Conservative Machine
Translation
保守な機械翻訳
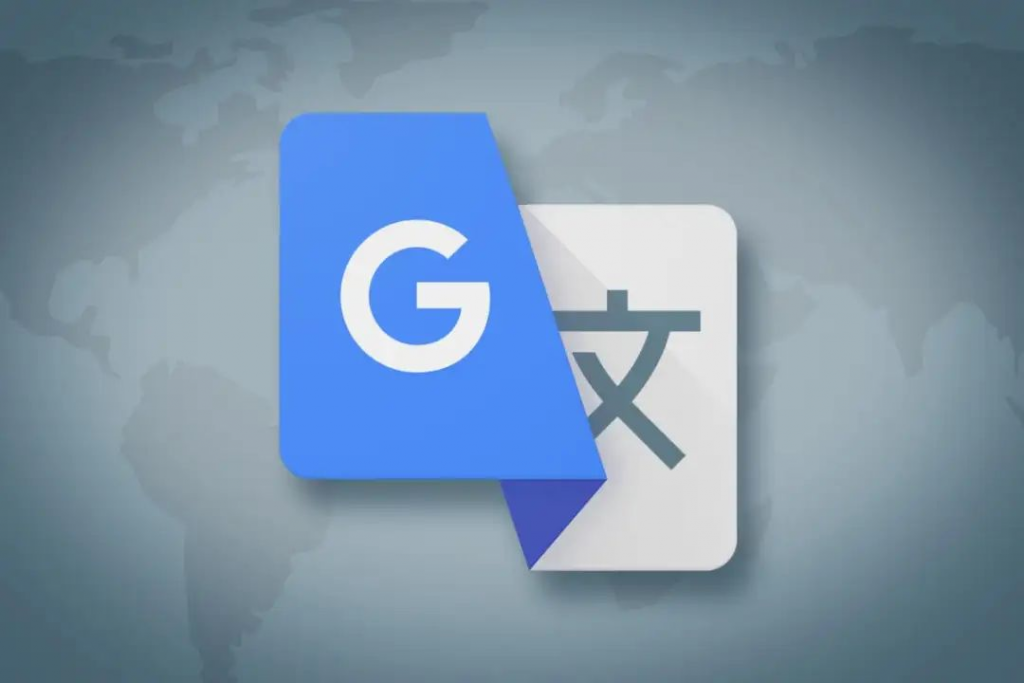
The results revealed that MT is more “conservative” than HT, exhibiting less morphosyntactic diversity, more convergent patterns, and more one-to-one alignments. They also observed that MT tends to be less similar to HT when the source has less common structures.
結果は、MTがHTよりも「保守的」であり、形態句法の多様性が少なく、収束パターンが多く、1対1のアラインメントが多いことを示しています。また、ソースがより一般的でない構造を持つ場合、MTはHTに比べて類似性が低くなる傾向があることも観察されました。
The authors attributed this discrepancy to the use of beam search, which biases MT towards more convergent patterns. This bias is most prominent when convergent patterns appear frequently — around 50% of the time — in the training data. “This could be because the model has seen the pattern enough to assign it substantial probability mass, but there is still enough uncertainty that humans will frequently choose other patterns,” said the authors.
著者は、この差異をビームサーチの使用に帰因しました。これにより、MTはより収束するパターンに偏ります。このバイアスは、収束パターンが頻繁に現れる場合に最も顕著です – 訓練データの約50%の時間です。著者は、「これは、モデルがパターンを十分に見て、それに相当する確率を大量に割り当てたためかもしれませんが、人間が頻繁に他のパターンを選択する十分な不確実性がまだあるためです」と述べています。
Moreover, frequencies of convergent patterns in MT increase even when they are uncommon in HT, suggesting perhaps a more inherent structural bias in current MT architectures.
さらに、MTにおける収束パターンの頻度は、HTでは一般的でない場合でも増加し、現在のMTアーキテクチャにおけるより固有の構造的バイアスを示唆しています。
Lastly, they investigated how the presence of morphosyntactic divergence in HT might affect MT quality and found that, for a majority of morphosyntactic divergences, their presence in HT is correlated with decreased MT performance, presenting a greater challenge for MT systems.
最後に、彼らはHTにおける形態句法の発散の存在がMTの品質にどのように影響するかを調査しました。そして、多くの形態句法の発散に対して、その存在がMTの性能低下と相関しており、MTシステムにとってより大きな挑戦を提供していることがわかりました。
The authors emphasized that “this is the first work to present the comparative perspective of HT vs MT in such fine granularity covering thousands of morphosyntactic constructions,” and expressed their interest in applying the same analysis to large language model (LLM)-based MT systems and see if and how the LLM translations differ from those produced by traditional MT models.
著者は、「これが数千の形態句法構造をカバーするこのような細かい粒度でHTとMTの比較的視点を提示する最初の作品である」と強調し、同じ分析を大規模言語モデル(LLM)ベースのMTシステムに適用し、LLM翻訳が伝統的なMTモデルによって生成されたものとどのように異なるかを見ることに興味を示しました。
効率的で正確なリアルタイム翻訳ツール— Felo 瞬訳

Felo 瞬訳とは何ですか?
Felo 瞬訳は、GPT-4エンジンとRRTテクノロジーを搭載したAI同時通訳アプリです。英語、スペイン語、フランス語、ドイツ語、ロシア語、中国語、アラビア語、日本語など15以上の外国語の音声を迅速かつ正確に翻訳できます。オリジナルと翻訳されたテキストのダウンロードをサポートし、正確な表現や発音の学習を支援します。大規模な言語モデルであるChatGPTは、舞台の情熱、表現、および劇的効果を正確に伝え、観客が異なる言語文化からもたらされる興奮を十分に理解し楽しむことができます。
Felo Translatorは、同時通訳者にどのように支援できますか?
Felo 瞬訳は、同時通訳の初心者をサポートし、ノートを取り残さず、専門用語の翻訳をより正確に行うことで問題を解決します。
同時通訳は複雑で高度な技術を要する仕事であり、通訳者はしっかりした言語スキル、豊富な専門知識、そして良好なチームワーク精神を持つ必要があります。継続的な学習と自己の翻訳能力の向上のみが、この重要な翻訳タスクに適任であり、国際コミュニケーションの円滑な進展に貢献できます。